Dear Reader,
I decided to write quickly about 6 programs/books I took: AI Programming with Python (Udacity), Google Cloud Platform Architecting (Coursera), Creating Kivy Apps by Dusty Philips, Linux Kernel Development by Robert Love, Terraform: Up and Running by Yevgeniy Brikman and the Design of Everyday Things by Donald A. Norman.
Udacity: AI Programming with Python
This is the entry-level course in AI for Udacity. Udacity is an online course-ware provider that focuses on providing content on cutting-edge topics: AI, Deep Learning, Self-driving cars and Node/React. It also has classes on data analysis and marketing. AI program starts by teaching you python, brushing up on some linear algebra and finishing off with 4 sections on AI: Two theoretical units on gradient descent and neural networks, one on PyTorch and a final project that classifies flowers. PyTorch is Facebook’s AI framework built in Python.
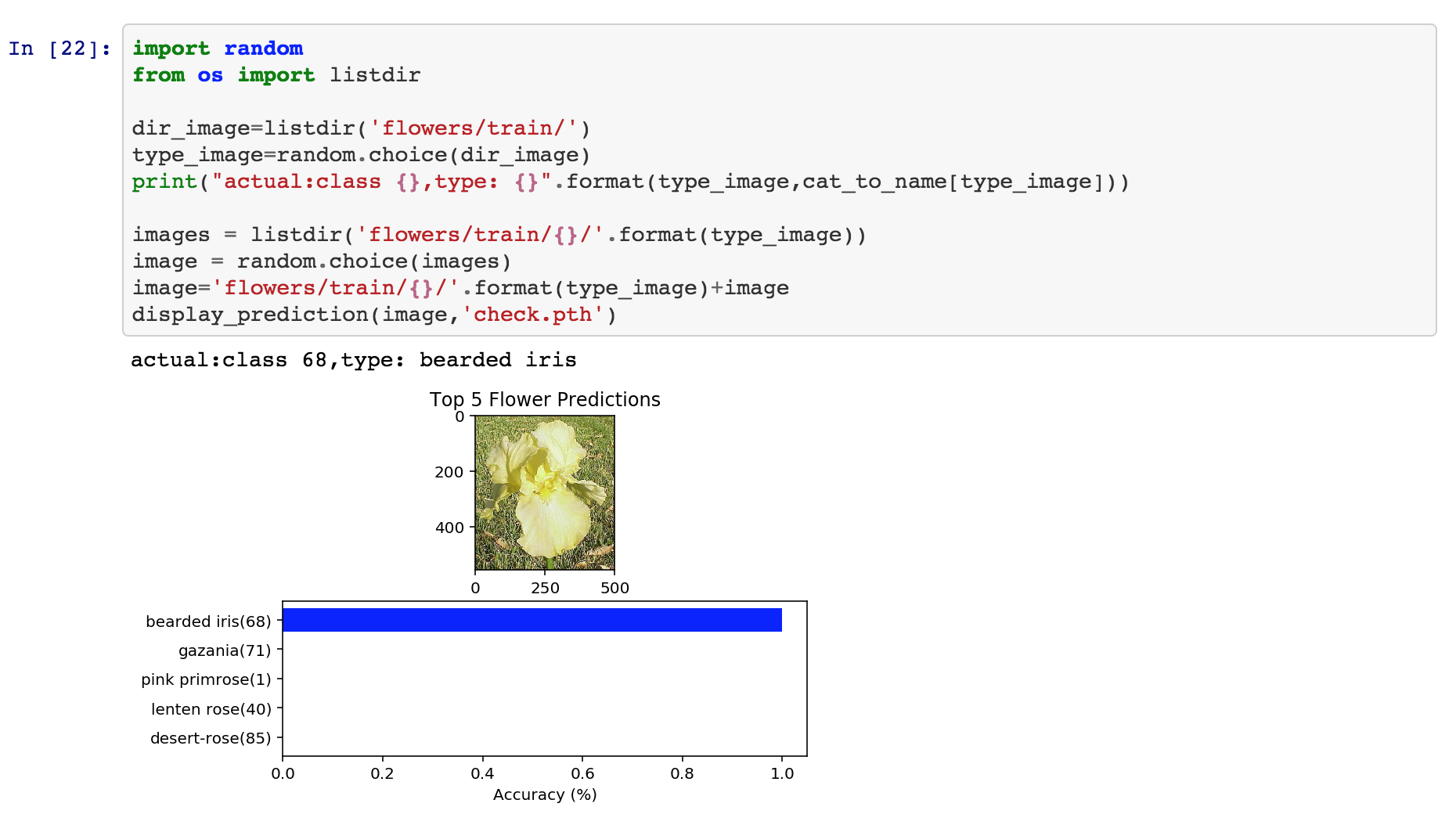
AI Class
The course is well prepared overall and should be manageable for someone with intermediate programming skills. Best in Python. It’s a bit on the expensive side for online material: $500-$1000, but Udacity tends to make up for the price tag with more interesting content and topics. Overall the quality is good. I’ve heard FastAI is a free alternative.
My Repo: https://github.com/Silber8806/udacity-AI-programming-with-python
Coursera: Architecting with Google Cloud Platform
Google Cloud Platform, GCP, is googles answer to AWS, Amazon Web Services. Overall, the platform has many of the same services as Amazon including Cloud Functions, which looks to be AWS Lambda for Google Cloud. The only glaring issues I’ve noticed so far: 1. No equivalent to SNS for e-mail and some UI issues in the console concerning Google Cloud Storage (S3). The first part is painful in that Google by default blocks the smtp ports forcing you to use a 3rd party provider (with limited support in stackdriver).
Google outsourced most of it’s training for GCP to coursera. The courses are broken into 6 parts. The first course covers all of the services on a 40,000 foot level, 4 courses proceeding this are in-depth technical reviews of virtual machines, networking, storage, database technologies, container services and autoscaling solutions. It also includes a host of managed services including the very neat sounding: “Spanner”, a supposedly horizontally scaling RDBMS service.
The last course, Reliable Cloud Infrastructure: Design and Process, was the most interesting. It provides a practical overview of how to construct infrastructure as well as going over Google’s philosophy on designing scalable software.
Something that really stood out about this program is the labs. They provide you a temporary Google Cloud Account for your tutorials. You get to practice on the actual Google console and shell prompts. This program is reasonably priced at $49.99 per month. You can take the material without labs for free, but I’d recommend the labs!
Creating Apps in Kivy:
Kivy is Python’s Mobile Application development framework. It’s used to make cross-platform applications for: Android, IPhone and Ipads. It’s something I’ve wanted to try out for a while just out of curiosity. I picked up Creating Apps in Kivy by Dusty Phillips, a book on the subject.
The book covers two applications: A weather application using an open weather api and a video game involving spaceships. The weather app takes the block of the book with significant amount of time spent on UI and UI interactions. Kivy generally is split into two files: a main file in python that is event-driven and a .kv file that describes the component layout. A single chapter is dedicated to graphics. The best part of the graphics section was developing animated snowflakes that fell from a single component. The book also covers databases and advanced UI components like a carousel.
Overall, I’m happy with the quality of this book with the exception of 2 things. It was hard to read the indentation between pages and there were a few chapters where I had to add imports or features he didn’t mention to get the examples to run. For this reason, I’d recommend being somewhat well versed in Python before attempting the sample projects in the book.
Github Repository: https://github.com/Silber8806/kivy_tutorial
Linux Kernel Development (3rd Edition) by Robert Love:
I started reading this book after my colleague at Salesforce.com, a DevOps Engineer, began talking to me more about the Linux Kernel and strange behaviors in Bash. I read a previous book to get a better understanding of the OS and am really happy that I skimmed through this book.
Linux Kernel Development covers the Linux source code by going through different subsystems and explaining how it works. It’s a deep-dive into things like: process management, memory, I/O, Virtual File System, Cacheing, Timesharing… It’s inspirational to see how much thought has gone into the Operating System and how many improvements are mentioned. You really feel like you are standing on the shoulders of giants after reading this book.
Linux Kernel Development feels like a technical reference and I’d recommend reading a book on Operating Systems before getting too deep into it (or taking courses in C). I skimmed through this book, because I use Linux daily and feel a bit clueless about what is happening under the covers and wanted to learn more about it.
Terraform: Up and Running by Yevgeniy Brikman:
Terraform: Up and Running covers… Terraform, the cloud provisioning software by HCL corp. . Terraform is a framework for deploying IT services to AWS, GCP, Azure and Digital Oceans in an automated fashion.
Terraform is cool in that you write your infrastructure as a series of templates. These templates are in the form of a declarative language called HCL, which describes your cloud infrastructure typically in a 1-to-1 fashion with the services you have deployed. What’s special about Terraform is that after you apply a HCL template to your cloud environment, it writes the current state into a file (immutable data structure). Every additional change begins with your last state and modifies it. A lot of devops systems are not aware of their previous configurations. Terraform reminds me a little of Redux, but applied to Infrastructure instead of web development (I might be wrong).
Things I noticed from the book. They suggested deploying these state files to S3, expect strong concurrency controls around the Terraform library and advice that you don’t mix other devops tools with Terraform. That way if a user manual creates an account that Terraform isn’t aware of, it won’t create an error (since that manually created user isn’t part of Terraform’s state). It seems Terraform works best as an isolated service and the “main” provisioning tool for a specific subspace of your infrastructure.
I skimmed through this book as I was interested in Terraform and have heard of a lot of adoptions in industry of this technology. I didn’t get to test the code in this book and can’t really speak about it.
The Design of Everyday Things by Donald Norman:
The Design of Everyday Things by Donald A. Norman is a book about usability and user design. It’s the book that I’ve been listening to on Audible, an audio book app by Amazon.com.
The book goes over the design of everyday things: ovens, doors, cars, computers, phone systems, radios and details both design flaws and positive things about different objects. He focuses a lot on understanding differences between knowledge in the world vs in the mind, how we map controls (buttons etc) to actions and how overloading them can lead to confusion and how to constrict user settings to prevent too many options flooding the users consciousness.
He’s a big proponent of mapping controls to physical aspects of the world. For example, a car seat control should move the seat forward if a switch is moved forward and backwards if a switch is flipped backwards. A switch shouldn’t move forward and backwards if a button is pressed left or right as that will confuse a user. He also favors designs were cues exist in the world that indicate what to do and where each control (button) does one and only one thing. A button on a watch should only turn off the alarm not adjust the alarm or answer phone calls as well. If these aspects of designs can’t be applied, he suggests standardization across an industry. A water facet for example should have cold water knob always to the right of the hot water knob.
This is a great book. I would suggest reading it on Kindle or as a paperback book. My big issue with Audible and Audio books in general is that I typically listen to them while walking or multi-tasking. That means I lose focus on certain sections (last 2 chapters). Overall, I really enjoyed the content I heard from this book.
Best,
Chris